

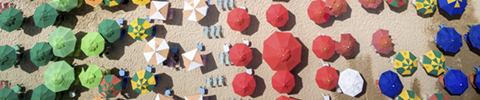
Resources for CRTs
Cluster randomised trials (CRTs) differ from individually randomised trials by the presence of correlation between observations within the same cluster. This has implications for the design and analysis since this clustering must be taken into account. Moreover, CRTs are often more prone to bias so this needs to be assessed and reported carefully.
This page provides resources to help trialists and researchers to conduct a CRT following existing guidelines and using the most recent methodological developments.
See What is a CRT? for several textbooks that give an excellent overview of the different stages of the design, conduct, analysis and reporting of CRTs, along with practical examples.
Resources below are separated into sections on Design, Analysis and Reporting.
Design: Introduction
This page provides resources to help with designing a CRT. It covers the following aspects: general information, pragmatic trials, pilot and feasibility studies, ethics, methods to identify and prevent bias at the design stage, randomisation methods, and sample size.
Design: general information
Turner et al. reviewed the recent developments in the design of CRTs:
-
Turner EL, Li F, Gallis JA, Prague M, Murray DM. Review of Recent Methodological Developments in Group-Randomized Trials: Part 1—Design. Am J Public Health. 2017 Jun;107(6):907–15
Recommendations have been proposed to design efficient CRTs:
-
Hemming K, Eldridge S, Forbes G, Weijer C, Taljaard M. How to design efficient cluster randomised trials. BMJ. 2017;358:j3064
Design: Pragmatic trials
Many CRTs are pragmatic in that they are aiming to test the effectiveness of interventions as applied in the real world rather than ideal conditions. Although not developed for CRTs only, the Precis-2 tool should be used to design more pragmatic trials:
-
Loudon K, Treweek S, Sullivan F, Donnan P, Thorpe KE, Zwarenstein M. The PRECIS-2 tool: designing trials that are fit for purpose. British Medical Journal. 2015;350:h2147
Design: Pilot and feasibility studies
Pilot and feasibility studies are often useful before conducting a main CRT. This is because of the added complexity in CRTs compared to individually randomised trials, meaning it is important to ensure that conducting a CRT is feasible before conducting the main trial:
-
Chan CL, Leyrat C, Eldridge SM. Quality of reporting of pilot and feasibility cluster randomised trials: a systematic review. BMJ Open. 2017;7(11)
This website gives more information on pilot and feasibility studies.
Design: Ethics
In individually randomised trials, consent to participate usually covers the willingness to be randomised, to be subject to the intervention being tested, and to be contacted for follow-up and for data collection. In a CRT these aspects may be separated and opting out of the intervention at an individual level may not be possible. CRTs therefore have unique features that complicate the application of standard ethical guidelines for research.
The Ottawa Statement was developed to provide detailed guidance to researchers, research ethics committees and regulators:
-
Taljaard M, Weijer C, Grimshaw JM, Eccles MP. The Ottawa Statement on the ethical design and conduct of cluster randomised trials: précis for researchers and research ethics committees. British Medical Journal. 2013;346:f2838
The following paper provides a practical and useful framework to guide researchers and research ethics committees through consent issues in CRTs:
-
Nix HP, Weijer C, Brehaut J, Forster D, Goldstein CE, Taljaard M. Informed consent in cluster randomised trials: a guide for the perplexed. BMJ Open. 2021;11:e054213
Design: Methods to identify and prevent bias
CRTs might be prone to bias due to the procedures surrounding patient recruitment. A review of these biases and solutions to prevent them have been proposed:
-
Giraudeau B, Ravaud P. Preventing bias in cluster randomised trials. PLoS Medicine. 2009;6(5):e1000065
-
Eldridge S, Kerry S, Torgerson DJ. Bias in identifying and recruiting participants in cluster randomised trials: what can be done? British Medical Journal. 2009;339:b4006
-
Hahn S, Puffer S, Torgerson DJ, Watson J. Methodological bias in cluster randomised trials. BMC Medical Research Methodology. 2005;5(1):10.
-
Eldridge S, Ashby D, Bennett C, Wakelin M, Feder G. Internal and external validity of cluster randomised trials: systematic review of recent trials. British Medical Journal. 2008;336(7649):876-80
-
Eldridge S, Kerry S. Chapter 2.3: Selection and recruitment of participants to enhance internal validity (pages 35-43) in ‘A Practical Guide to Cluster Randomised Trials in Health Services Research’ Chichester, West Sussex John Wiley & Sons; 2012
-
DiazOrdaz K, Slowther A-M, Potter R, Eldridge S. Consent processes in cluster-randomised trials in residential facilities for older adults: a systematic review of reporting practices and proposed guidelines. BMJ Open. 2013;3(7)
-
Froud R, Eldridge S, Ordaz KD, Marinho VCC, Donner A. Quality of cluster randomized controlled trials in oral health: a systematic review of reports published between 2005 and 2009. Community Dentistry and Oral Epidemiology. 2012;40(s1):3-14
-
Puffer S, Torgerson D, Watson J. Evidence for risk of bias in cluster randomised trials: review of recent trials published in three general medical journals. BMJ. 2003;327(7418):785-9
Caille et al developed a graphical tool to help with identifying bias at the design (and reporting) stage:
-
Caille A, Kerry S, Tavernier E, Leyrat C, Eldridge S, Giraudeau B. Timeline cluster: a graphical tool to identify risk of bias in cluster randomised trials. British Medical Journal. 2016;354:j4291
This tool can be easily designed online.
The Cochrane Risk of Bias (RoB) tool is being extended to cluster randomised trials. A draft version of the tool and supporting information can be found on the RoB website.
Design: Randomisation methods
The design chapter in Eldridge and Kerry’s book provides a good introduction to randomisation methods in CRTs:
-
Eldridge S, Kerry S. ‘Chapter 5: Design’ (pages 74-98) in ‘A practical guide to cluster randomised trials in health services research’. Chichester, West Sussex: John Wiley & Sons; 2012.
For parallel CRTs, standard cluster randomisation does not always ensure baseline covariate balance between arms. Ivers et al. have described alternative randomisation techniques, including stratification and minimisation:
-
Ivers NM, Halperin IJ, Barnsley J, Grimshaw JM, Shah BR, Tu K, et al. Allocation techniques for balance at baseline in cluster randomized trials: a methodological review. Trials. 2012;13(1):120
Li et al. have investigated the performance of constrained randomisation:
-
Li F, Turner EL, Heagerty PJ, Murray DM, Vollmer WM, DeLong ER. An evaluation of constrained randomization for the design and analysis of group‐randomized trials with binary outcomes. Statistics in Medicine. 2017;36(24):3791-806
Pseudo cluster-randomisation is also an interesting strategy to deal with selection bias and contamination, as well as the “best allocation” method:
-
Teerenstra S, Melis RJF, Peer PGM, Borm GF. Pseudo cluster randomization dealt with selection bias and contamination in clinical trials. Journal of Clinical Epidemiology. 2006;59(4):381-6
-
de Hoop E, Teerenstra S, van Gaal BGI, Moerbeek M, Borm GF. The “best balance” allocation led to optimal balance in cluster-controlled trials. J Clin Epidemiol. 2012 février;65(2):132–7
Various practical resources have been produced. See Design – Tools and software.
Design: Sample size
Clustering must be accounted for when estimating the sample size. For an overview of sample size formulas and practical recommendations:
-
Rutterford C, Copas A, Eldridge S. Methods for sample size determination in cluster randomized trials. International Journal of Epidemiology. 2015;44(3):1051-67
-
Donner A, Birkett N, Buck C. Randomization By Cluster: Sample Size Requirements And Analysis. American Journal of Epidemiology. 1981;114(6):906-14
-
van Breukelen GJP, Candel MJJM. Calculating sample sizes for cluster randomized trials: We can keep it simple and efficient! Journal of Clinical Epidemiology. 2012;65(11):1212-8
Specific methods have been proposed to estimate the sample size in the following situations:
Fixed number of clusters
-
Hemming K, Girling AJ, Sitch AJ, Marsh J, Lilford RJ. Sample size calculations for cluster randomised controlled trials with a fixed number of clusters. BMC Medical Research Methodology. 2011;11(1):102
Different sized clusters
-
Eldridge SM, Ashby D, Kerry S. Sample size for cluster randomized trials: effect of coefficient of variation of cluster size and analysis method. International Journal of Epidemiology. 2006;35(5):1292-300
Three-level CRTs
-
Teerenstra S, Lu B, Preisser JS, Achterberg Tv, Borm GF. Sample Size Considerations for GEE Analyses of Three‐Level Cluster Randomized Trials. Biometrics. 2010;66(4):1230-7
Time-to-event outcomes
-
Jahn‐Eimermacher A, Ingel K, Schneider A. Sample size in cluster‐randomized trials with time to event as the primary endpoint. Statistics in Medicine. 2013;32(5):739-51
Expected attrition/missing data
-
Taljaard M, Donner A, Klar N. Accounting for expected attrition in the planning of community intervention trials. Statistics in Medicine. 2007;26(13):2615-28
Various practical resources have been produced for calculating sample size in CRTs. See Design – Tools and software.
Design: Tools and software
Randomisation resources
Practical resource in R for undertaking allocation concealed blocked randomisation:
SAS macro for constrained covariate randomisation of cluster randomised and unstratified stepped-wedge designs:
-
ccrsw-sas – SAS (Github)
Stata command to perform covariate-constrained randomisation for achieving baseline balance in CRTs:
Sample size resources
Sample size/power calculations for CRTs in Stata:
-
clsampsi (for CRTs with differential clustering)
-
samplesize (for any type of trial design)
Sample size/power calculations for CRTs in R:
Sample size/power calculations for CRTs in SAS:
-
power_calculator – SAS (Github) (with survival outcome)
Online sample size calculator for CRTs:
Stand-alone program to perform power analysis for multilevel data: (Moerbeek M, Teerenstra S. Power Analysis of Trials with Multilevel Data. Boca Raton: Chapman and Hall/CRC 2015)
Design: Intracluster correlation coefficient
For an overview of the intra-cluster correlation coefficient, see chapter 8 of Eldridge and Kerry’s book:
-
Eldridge S, Kerry S. Chapter 8: The intra-cluster correlation coefficient (pages 172-217) in ‘A practical guide to cluster randomised trials in health services research’. Chichester, West Sussex: John Wiley & Sons; 2012.
Sample size calculations for CRTs requires postulating a value for the intracluster correlation coefficient (ICC) (see Correlations in CRTs). One may, therefore, consider conducting a pilot study to estimate the ICC.
During analysis, computer packages estimate the intracluster correlation coefficient (ICC). Potential methods depend on the outcome:
-
Donner A. A review of inference procedures for the intraclass correlation coefficient in the one-way random effects model. International Statistical Review. 1986;54(1):67-82
-
Campbell MK, Grimshaw JM, Piaggio G. ‘Intraclass Correlation Coefficient with Emphasis on Clinical Trials’. Wiley StatsRef: Statistics Reference Online: John Wiley & Sons; 2014
-
Eldridge SM, Ukoumunne OC, Carlin JB. The Intra‐Cluster Correlation Coefficient in Cluster Randomized Trials: A Review of Definitions. International Statistical Review. 2009;77(3):378-94
-
Ridout MS, Demétrio CGB, Firth D. Estimating Intraclass Correlation for Binary Data. Biometrics. 1999;55(1):137-48
Giraudeau cautions that clusters from different groups should not be mixed when estimating the ICC:
-
Giraudeau B. Model mis‐specification and overestimation of the intraclass correlation coefficient in cluster randomized trials. Statistics in Medicine. 2006;25(6):957-64
Methods to estimate confidence intervals for the ICC are described in:
-
Zou G, Donner A. Confidence Interval Estimation of the Intraclass Correlation Coefficient for Binary Outcome Data. Biometrics. 2004;60(3):807-11
-
Ukoumunne OC, Davison AC, Gulliford MC, Chinn S. Non‐parametric bootstrap confidence intervals for the intraclass correlation coefficient. Statistics in Medicine. 2003;22(24):3805-21
-
Swiger L, Harvey WR, Everson D, Gregory K. The variance of intraclass correlation involving groups with one observation. Biometrics. 1964;20(4):818-26
However, Eldridge et al. suggest that pilot studies alone will usually be too small to estimate parameters required for estimating a sample size for a main CRT:
-
Eldridge SM, Costelloe CE, Kahan BC, Lancaster GA, Kerry SM. How big should the pilot study for my cluster randomised trial be? Statistical Methods in Medical Research. 2016;25(3):1039-56
-
Eldridge S, Kerry S. ‘Chapter 8.3: Choosing the ICC for use in sample size calculations’ (pages 179-185) in ‘A practical guide to cluster randomised trials in health services research’. Chichester, West Sussex: John Wiley & Sons; 2012.
Instead, reviews of ICC values observed in published trials, and especially work on patterns in ICCs, may help in choosing an appropriate value:
-
Turner RM, Thompson SG, Spiegelhalter DJ. Prior distributions for the intracluster correlation coefficient, based on multiple previous estimates, and their application in cluster randomized trials. Clinical Trials. 2005;2(2):108-18
-
Adams G, Gulliford MC, Ukoumunne OC, Eldridge S, Chinn S, Campbell MJ. Patterns of intra-cluster correlation from primary care research to inform study design and analysis. Journal of Clinical Epidemiology. 2004;57(8):785-94
-
Preisser JS, Reboussin BA, Song E-Y, Wolfson M. The Importance and Role of Intracluster Correlations in Planning Cluster Trials. Epidemiology. 2007;18(5):552-60
Analysis: Introduction
The main consideration in the analysis of CRTS, beyond those of the parallel individually randomised trial, is to account for the clustered structure of the data. Observations in the same cluster are likely to be more similar to one another than observations in different clusters and this needs to be accounted for in the analysis.
We present some general information, followed by specific methods including cluster summary analyses, random effect methods/generalised linear mixed effect models, and generalised estimating equations (GEE). Methods may vary depending on the type of outcome.
Trialists may need to consider whether methods are appropriate if they have a small number of clusters. There are also methods for dealing with missing data and there are causal inference methods in the situation of non-compliance or indirect effects of the intervention.
If performing a meta-analysis, there are specific methods to include CRT results in meta-analyses.
Finally Bayesian methods have been developed as an alternative framework to the frequentist methods of analysing CRTs.
Analysis: General information
The analysis chapter in Eldridge and Kerry’s book provides a good introduction to analysis in CRTs with lots of examples:
-
Eldridge S, Kerry S. ‘Chapter 6: Analysis’ (pages 99-136) in ‘A practical guide to cluster randomised trials in health services research’. Chichester, West Sussex: John Wiley & Sons; 2012.
Turner et al. reviewed the recent developments in the analysis of CRTs:
-
Turner EL, Prague M, Gallis JA, Li F, Murray DM. Review of Recent Methodological Developments in Group-Randomized Trials: Part 2—Analysis. American Journal of Public Health. 2017;107(7):1078-86
The following provide a comparison of the various analysis methods:
-
Peters TJ, Richards SH, Bankhead CR, Ades AE, Sterne JAC. Comparison of methods for analysing cluster randomized trials: an example involving a factorial design. International Journal of Epidemiology. 2003;32(5):840-6
-
Austin PC. A comparison of the statistical power of different methods for the analysis of cluster randomization trials with binary outcomes. Statistics in Medicine. 2007;26(19):3550-65
Analysis: Cluster summary
In cluster summary analyses, regression models are fitted on cluster-level summaries of the outcome (e.g. the cluster mean). However, if cluster sizes are variable, clusters must be weighted accordingly.
-
Hayes RJ, Moulton LH. Cluster randomised trials. Boca Raton: CRC Press; 2017
-
Kerry SM, Bland JM. Analysis of a trial randomised in clusters. British Medical Journal. 1998;316(7124):54
-
Kerry SM, Bland JM. Trials which randomize practices I: how should they be analysed? Family Practice. 1998;15(1):80-3
Analysis: Random effect methods/generalised linear mixed effect models
Adjusting for baseline covariates using cluster summary methods is not straightforward and therefore individual-level regression models are often preferred. Using generalised linear mixed-effect models, or random effects models, allow the estimation of a cluster-specific random effect.
Mixed effects models are described in chapters 6.3.3.4 and 6.3.3.9 of Eldridge and Kerry’s book, for continuous and binary outcomes, respectively:
-
Eldridge S, Kerry S. ‘Chapter 6: Analysis’ (pages 99-136) in ‘A practical guide to cluster randomised trials in health services research’. Chichester, West Sussex: John Wiley & Sons; 2012.
The following article also discusses generalised linear mixed models:
-
Mcculloch CE, Neuhaus JM. ‘Generalized Linear Mixed Models Based in part on the article “Generalized linear mixed models” by Charles E. McCulloch, which appeared in the Encyclopedia of Environmetrics’. Encyclopedia of Environmetrics: John Wiley & Sons; 2013
Analysis: Generalised estimating equations
Generalised estimating equations (GEEs) estimate a population-average effect while accounting for the intracluster correlation.
Generalised estimating equations are described in chapters 6.3.3.3 and 6.3.3.8 of Eldridge and Kerry’s book, for continuous and binary outcomes, respectively:
-
Eldridge S, Kerry S. ‘Chapter 6: Analysis’ (pages 99-136) in ‘A practical guide to cluster randomised trials in health services research’. Chichester, West Sussex: John Wiley & Sons; 2012.
The following article also discusses generalised estimating equations:
-
Dahmen G, Ziegler A. Generalized Estimating Equations in Controlled Clinical Trials: Hypotheses Testing. Biometrical Journal. 2004;46(2):214-32
Analysis: Type of outcome
The following papers discuss the analysis of CRTs when considering a specific outcome:
Continuous outcomes
-
Feng Z, Mclerran D, Grizzle J. A Comparison Of Statistical Methods For Clustered Data Analysis With Gaussian Error. Statistics in Medicine. 1996;15(16):1793-806
Binary outcomes
-
Omar RZ, Thompson SG. Analysis of a cluster randomized trial with binary outcome data using a multi‐level model. Statistics in Medicine. 2000;19(19):2675-88
-
Austin PC. A comparison of the statistical power of different methods for the analysis of cluster randomization trials with binary outcomes. Statistics in Medicine. 2007;26(19):3550-65
-
Bennett S, Parpia T, Hayes R, Cousens S. Methods for the analysis of incidence rates in cluster randomized trials. International Journal of Epidemiology. 2002;31(4):839-46
-
Zou G, Donner A. Extension of the modified Poisson regression model to prospective studies with correlated binary data. Statistical Methods in Medical Research. 2013;22(6):661-70
Time-to-event outcomes
-
Austin PC. A Tutorial on Multilevel Survival Analysis: Methods, Models and Applications. International Statistical Review. 2017;85(2):185-203
Analysis: Small number of clusters
The implementation of the approaches above can be challenging when a small number of clusters are randomised:
-
Leyrat C, Morgan KE, Leurent B, Kahan BC. Cluster randomized trials with a small number of clusters: which analyses should be used? International Journal of Epidemiology. 2018;47(1):321-31
Analysis: Missing data
Missing outcomes should be assessed and reported in the analysis of CRTs. One may choose to do a sensitivity analysis. Multiple imputation performs well to address the missing at random problem (where missingness is not specifically related to the missing information but is related to some of the observed data), both for continuous and binary outcomes:
-
Taljaard M, Donner A, Klar N. Imputation Strategies for Missing Continuous Outcomes in Cluster Randomized Trials. Biometrical Journal. 2008;50(3):329-45
-
Caille A, Leyrat C, Giraudeau B. A comparison of imputation strategies in cluster randomized trials with missing binary outcomes. Statistical Methods in Medical Research. 2016;25(6):2650-69
-
Díaz-Ordaz K, Kenward MG, Cohen A, Coleman CL, Eldridge S. Are missing data adequately handled in cluster randomised trials? A systematic review and guidelines. Clinical Trials. 2014;11(5):590-600
In theory these methods allow us to account for missing data on covariates as well, but focus on the outcome (which is usually the challenge in trials since baseline data are usually well recorded).
The following practical resources are for performing multiple imputation accounting for the clustered nature of the data in R:
Using joint modelling in R
-
Quartagno M, Carpenter J. jomo: Multilevel Joint Modelling Multiple Imputation. The Comprehensive R Archive Network; 2016
Using chained equations in R
-
Audigier V, Resche-Rigon M. micemd: Multiple Imputation by Chained Equations with Multilevel Data. 2018
Analysis: Causal inference
Because CRTs are often pragmatic trials, they allow the estimation of the effectiveness of the intervention, that is, the effect of the intervention in real-life situations, including non-compliance or indirect effects of the intervention.
However, it is possible to estimate a complier average causal effect in CRTs, that is, the effect of the intervention based on compliers. Compliers are patients in the intervention group who receive the intervention, and patients in the control group who do not receive the intervention but would have received the intervention had they been randomised to it.
-
Jo B, Asparouhov T, Muthén BO. Intention‐to‐treat analysis in cluster randomized trials with noncompliance. Statistics in Medicine. 2008;27(27):5565-77
-
Schochet PZ, Chiang H. Estimation and Identification of the Complier Average Causal Effect Parameter in Education RCTs (NCEE 2009-4040). Washington, DC: National Center for Education Evaluation and Regional Assistance, Institute of Education Sciences, U.S. Department of Education; 2009
It is also possible to measure the indirect effect through mediation analysis:
-
Hox J, Moerbeek M, Kluytmans A, Van De Schoot R. Analyzing indirect effects in cluster randomized trials. The effect of estimation method, number of groups and group sizes on accuracy and power. Frontiers in Psychology. 2014;5(78)
Analysis: CRTs in meta-analyses
Cluster and individually randomised trials are often meta-analysed together in systematic reviews. Specific methods to include CRT results in meta-analyses can be found in:
-
Higgins JPT, Green S. ‘Chapter 16’. Cochrane Handbook for Systematic Reviews of Interventions. Chichester, West Sussex: John Wiley & Sons; 2011
-
Donner A, Piaggio G, Villar J. Statistical methods for the meta-analysis of cluster randomization trials. Statistical Methods in Medical Research. 2001;10(5):325-38
The Cochrane Risk of Bias (RoB) tool is being extended to cluster randomised trials. This tool can be used to assess the risk of bias in studies in a systematic review. A draft version of the tool and supporting information can be found on the RoB website.
Analysis: Bayesian methods
Bayesian methods have also been developed as an alternative framework to analyse CRTs:
-
Spiegelhalter DJ. Bayesian methods for cluster randomized trials with continuous responses. Statistics in Medicine. 2001;20(3):435-52
-
Turner RM, Omar RZ, Thompson SG. Bayesian methods of analysis for cluster randomized trials with binary outcome data. Statistics in Medicine. 2001;20(3):453-72
Reporting: Introduction
Adequate reporting of the trial results is essential to ensure transparency and reproducibility of findings. This page provides resources to help with the reporting of CRTs. It covers three aspects: guidelines for reporting, tools and evaluation of the risk of bias, and considerations for complex interventions.
Reporting: Guidelines
The Consolidated Standards of Reporting Trials (CONSORT) statement, first published in 1996 and subsequently updated includes a checklist of items that should be included in the trial report. Initially developed for individually randomised trials, an extension for CRTs is available:
-
Campbell MK, Piaggio G, Elbourne DR, Altman DG. Consort 2010 statement: extension to cluster randomised trials. British Medical Journal. 2012;345:e5661
It is now a requirement that those publishing in high impact journals follow the CONSORT checklist. This checklist can be used along with other relevant extensions, such as the extension for pilot trials or non-inferiority trials. All the supporting documents are freely available on the CONSORT website.
Campbell et al. also provide recommendations for the reporting of the intracluster correlation coefficient in CRTs:
-
Campbell MK, Grimshaw JM, Elbourne DR. Intracluster correlation coefficients in cluster randomized trials: empirical insights into how should they be reported. BMC Medical Research Methodology. 2004;4(1):9
Reporting: Current standard
Several systematic reviews showed that the current reporting of the results of CRTs is suboptimal in various contexts. Here we list a selection of these:
-
Chan CL, Leyrat C, Eldridge SM. Quality of reporting of pilot and feasibility cluster randomised trials: a systematic review. BMJ Open. 2017;7(11)
-
Jarvis C, Di Tanna GL, Lewis D, Alexander N, Edmunds WJ. Spatial analysis of cluster randomised trials: a systematic review of analysis methods. Emerging Themes in Epidemiology. 2017;14(1):12
-
Tokolahi E, Hocking C, Kersten P, Vandal AC. Quality and Reporting of Cluster Randomized Controlled Trials Evaluating Occupational Therapy Interventions: A Systematic Review. OTJR: Occupation, Participation and Health. 2016;36(1):14-24
-
Fiero MH, Huang S, Oren E, Bell ML. Statistical analysis and handling of missing data in cluster randomized trials: a systematic review. Trials. 2016;17(1):72
-
Díaz-Ordaz K, Kenward MG, Cohen A, Coleman CL, Eldridge S. Are missing data adequately handled in cluster randomised trials? A systematic review and guidelines. Clinical Trials. 2014;11(5):590-600
-
Ivers NM, Taljaard M, Dixon S, Bennett C, McRae A, Taleban J, et al. Impact of CONSORT extension for cluster randomised trials on quality of reporting and study methodology: review of random sample of 300 trials, 2000-8. BMJ. 2011;343
-
Eldridge SM, Ashby D, Feder GS, Rudnicka AR, Ukoumunne OC. Lessons for cluster randomized trials in the twenty-first century: a systematic review of trials in primary care. Clinical Trials. 2004;1(1):80-90
Reporting: Tools and evaluation of the risk of bias
The timeline cluster tool, which can also be used at the design stage, allows a graphical representation of the trial chronology, by intervention arm. It is flexible enough to describe a large variety of CRTs designs, such as cross-sectional, cross-over or longitudinal CRTs. It can also help with identifying the risk of bias surrounding the trial chronology:
-
Caille A, Kerry S, Tavernier E, Leyrat C, Eldridge S, Giraudeau B. Timeline cluster: a graphical tool to identify risk of bias in cluster randomised trials. British Medical Journal. 2016;354:j4291
A website providing the template and examples can be found here.
In the context of systematic reviews, risk of bias can also be assessed using the risk of bias tool (RoB.2), for which an extension for CRTs is being developed.
Reporting: Complex interventions
Complex interventions are common in CRTs. The following are guidelines for their reporting/development:
-
Medical Research Council. A framework for the development and evaluation of RCTs for complex interventions to improve health. London: MRC; 2000
-
Möhler R, Köpke S, Meyer G. Criteria for Reporting the Development and Evaluation of Complex Interventions in healthcare: revised guideline (CReDECI 2). Trials. 2015;16(1):204
-
Hoffmann TC, Glasziou PP, Boutron I, Milne R, Perera R, Moher D, et al. Better reporting of interventions: template for intervention description and replication (TIDieR) checklist and guide. BMJ. 2014;348
The following are graphical representations to describe complex interventions, often evaluated using CRTs:
-
Hooper R, Froud RJ, Bremner SA, Perera R, Eldridge S. Cascade diagrams for depicting complex interventions in randomised trials. British Medical Journal. 2013;347:f6681
-
Perera R, Heneghan C, Yudkin P. Graphical method for depicting randomised trials of complex interventions. BMJ. 2007;334(7585):127-9