

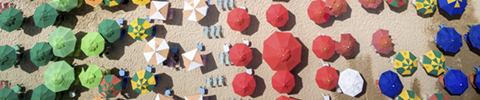
Resources for stepped-wedge CRTs
In a SW-CRT, not only is there correlation between observations within the same cluster, but the intervention effect is also confounded with changes in the outcome over time because the intervention condition is on average later in time than the control condition. This has implications for the design, analysis and reporting.
This page provides resources specific to conducting a SW-CRT and has some information for the related crossover CRT design, but the resources on CRTs may also be useful.
See What is a SW-CRT? for several recent publications giving an overview of issues in the design, conduct, analysis and reporting of SW-CRTs.
Resources below are separated into sections on Design, Analysis and Reporting.
Design: Introduction
This page provides resources to help with designing a SW-CRT. It may also be useful to look at the resources for designing CRTs, but here we focus on resources specific to conducting a SW-CRT. In particular, we cover: general information, pilot and feasibility studies, ethics, randomisation, sample size and selecting a design, expected inter-period correlation coefficient values, and the crossover design.
Many aspects of design depend on the choice of analysis, so you may want to refer to resources for analysing SW-CRTs.
Design: General information
-
Zhan Z, de Bock GH, van den Heuvel ER. Statistical methods for unidirectional switch designs: Past, present, and future. Stat Methods Med Res. 2018;27(9):2872-82
-
Taljaard M, Teerenstra S, Ivers NM, Fergusson DA. Substantial risks associated with few clusters in cluster randomized and stepped wedge designs. Clinical Trials. 2016;13(4):459-63
-
Copas AJ, Lewis JJ, Thompson JA, Davey C, Baio G, Hargreaves JR. Designing a stepped wedge trial: three main designs, carry-over effects and randomisation approaches. Trials. 2015;16(1):352
Design: Pilot and feasibility studies
Pilot and feasibility studies are often useful before conducting a main SW-CRT. This is because of the added complexity in SW-CRTs compared to individually randomised trials.
-
Kristunas CA, Hemming K, Eborall HC, Gray LJ. The use of feasibility studies for stepped-wedge cluster randomised trials: protocol for a review of impact and scope. BMJ Open. 2017;7(7)
Design: Ethics
The following papers discuss the ethical considerations when conducting a SW-CRT.
-
Prost A, Binik A, Abubakar I, Roy A, De Allegri M, Mouchoux C, et al. Logistic, ethical, and political dimensions of stepped wedge trials: critical review and case studies. Trials. 2015;16(1):351
-
de Hoop E, van der Tweel I, van der Graaf R, Moons KGM, van Delden JJM, Reitsma JB, et al. The need to balance merits and limitations from different disciplines when considering the stepped wedge cluster randomized trial design. BMC Medical Research Methodology. 2015;15(1):93
Design: Randomisation
All methods of randomisation that can be used for CRTs can also be used for SW-CRT. See Resources for designing CRTs.
This article discusses why it is important to randomise in a SW-CRT:
-
Hargreaves JR, Prost A, Fielding KL, Copas AJ. How important is randomisation in a stepped wedge trial? Trials. 2015;16(1):359
This article evaluates covariate constrained randomisation in SW-CRTs (see Resources for designing CRTs for an explanation of covariate constrained randomisation):
-
Chaussee EL, Dickinson LM, Fairclough DL. Evaluation of a covariate-constrained randomization procedure in stepped wedge cluster randomized trials. Contemporary Clinical Trials. 2021;105: p. 106409.
These articles give examples of using covariate constrained randomisation in a SW-CRT:
-
Diakite I, Mooring EQ, Velásquez GE, Murray MB. Novel Ordered Stepped-Wedge Cluster Trial Designs for Detecting Ebola Vaccine Efficacy Using a Spatially Structured Mathematical Model. PLOS Neglected Tropical Diseases. 2016;10(8):e0004866
-
Moulton LH, Golub JE, Durovni B, Cavalcante SC, Pacheco AG, Saraceni V, et al. Statistical design of THRio: a phased implementation clinic-randomized study of a tuberculosis preventive therapy intervention. Clinical Trials. 2007;4(2):190-9
Design: Sample size and selecting a design
To calculate the sample size required for a SW-CRT, you must make some assumptions about what your data will look like. In addition to the cluster size and ICC required for the sample size calculation of a parallel CRT, SW-CRTs require assumptions about changes in the correlation in your data over time. SW-CRTs cover a range of designs and the sample size is affected by including a baseline period, the number of sequences, and whether or not observations are collected in all clusters in all periods. Specific methods have been proposed to estimate the sample size and compare different designs in the following situations:
Assuming equal correlation within clusters across all periods with a cross-sectional design
(Note, this is a strong assumption that will usually be inappropriate, but the methods are simple. These methods may underestimate the required sample size):
-
Hu Y, Hoover DR. Non-randomized and randomized stepped-wedge designs using an orthogonalized least squares framework. Stat Methods Med Res. 2018;27(4):1202-18
-
Thompson JA, Fielding K, Hargreaves J, Copas A. The optimal design of stepped wedge trials with equal allocation to sequences and a comparison to other trial designs. Clinical Trials. 2017;14(6):639-47
-
Hemming K. Sample size calculations for stepped wedge trials using design effects are only approximate in some circumstances. Trials. 2016;17(1):234
-
Hemming K, Taljaard M. Sample size calculations for stepped wedge and cluster randomised trials: a unified approach. Journal of Clinical Epidemiology. 2016;69:137-46
-
Hemming K, Lilford R, Girling AJ. Stepped‐wedge cluster randomised controlled trials: a generic framework including parallel and multiple‐level designs. Statistics in Medicine. 2015;34(2):181-96
-
Liao X, Zhou X, Spiegelman D. A note on “Design and analysis of stepped wedge cluster randomized trials”. Contemporary clinical trials. 2015;45(Pt B):338-9
-
Lawrie J, Carlin JB, Forbes AB. Optimal stepped wedge designs. Statistics & Probability Letters. 2015;99:210-4
-
Woertman W, de Hoop E, Moerbeek M, Zuidema SU, Gerritsen DL, Teerenstra S. Stepped wedge designs could reduce the required sample size in cluster randomized trials. J Clin Epidemiol. 2013;66(7):752-8
-
Hussey MA, Hughes JP. Design and analysis of stepped wedge cluster randomized trials. Contemporary Clinical Trials. 2007;28(2):182-91
Assuming other correlation structures and accounting for cohort designs
-
Li F, Turner EL, Preisser JS. Optimal allocation of clusters in cohort stepped wedge designs. Statistics & Probability Letters. 2018;137:257-63
-
Li F, Turner EL, Preisser JS. Sample size determination for GEE analyses of stepped wedge cluster randomized trials. Biometrics. 2018. doi:10.1111/biom.12918
-
Heo M, Kim N, Rinke ML, Wylie-Rosett J. Sample size determinations for stepped-wedge clinical trials from a three-level data hierarchy perspective. Stat Methods Med Res. 2018;27(2):480-9
-
Kasza J, Hemming K, Hooper R, Matthews J, Forbes A. Impact of non-uniform correlation structure on sample size and power in multiple-period cluster randomised trials. Statistical Methods in Medical Research. 2017:0962280217734981
-
Matthews JNS, Forbes AB. Stepped wedge designs: insights from a design of experiments perspective. Statistics in Medicine. 2017;36(24):3772-90
-
Girling AJ, Hemming K. Statistical efficiency and optimal design for stepped cluster studies under linear mixed effects models. Statistics in Medicine. 2016;35(13):2149-66
-
Hooper R, Teerenstra S, Hoop E, Eldridge S. Sample size calculation for stepped wedge and other longitudinal cluster randomised trials. Statistics in Medicine. 2016;35(26):4718-28
-
Hooper R, Bourke L. Cluster randomised trials with repeated cross sections: alternatives to parallel group designs. British Medical Journal. 2015;350:h2925
Using simulation studies
(This is the most flexible method that can incorporate any design or correlation structures):
-
Landau S, Stahl D. Sample size and power calculations for medical studies by simulation when closed form expressions are not available. Statistical Methods in Medical Research. 2013;22(3):324-45
-
Baio G, Copas A, Ambler G, Hargreaves J, Beard E, Omar RZ. Sample size calculation for a stepped wedge trial. Trials. 2015;16(1):354
-
Hooper R, Teerenstra S, Hoop E, Eldridge S. Sample size calculation for stepped wedge and other longitudinal cluster randomised trials. Statistics in Medicine. 2016;35(26):4718-28
Designs with transition periods
-
Hemming K, Girling A. A menu-driven facility for power and detectable-difference calculations in stepped-wedge cluster-randomized trials. Stata Journal. 2014;14(2):363-80
Designing SW-CRTs with 3 arms
-
Lyons VH, Li L, Hughes JP, Rowhani-Rahbar A. Proposed variations of the stepped-wedge design can be used to accommodate multiple interventions. Journal of Clinical Epidemiology. 2017;86:160-7
-
Matthews JNS, Forbes AB. Stepped wedge designs: insights from a design of experiments perspective. Statistics in Medicine. 2017;36(24):3772-90
Unequal cluster size
-
Girling AJ. Relative efficiency of unequal cluster sizes in stepped wedge and other trial designs under longitudinal or cross-sectional sampling. Statistics in medicine. 2018.1-13.
-
Kristunas CA, Smith KL, Gray LJ. An imbalance in cluster sizes does not lead to notable loss of power in cross-sectional, stepped-wedge cluster randomised trials with a continuous outcome. Trials. 2017;18(1):109
-
Kristunas C, Morris T, Gray L. Unequal cluster sizes in stepped-wedge cluster randomised trials: a systematic review. BMJ Open. 2017;7(11)
Within-period/ vertical analysis
-
Hughes JP, Heagerty PJ, Xia F, Ren Y. Robust Inference for the Stepped Wedge Design. UW Biostatistics Working Paper Series 2018:Working Paper 424
-
Hayes RJ, Moulton LH. Cluster randomised trials. Boca Raton: CRC Press; 2017
-
Matthews JNS, Forbes AB. Stepped wedge designs: insights from a design of experiments perspective. Statistics in Medicine. 2017;36(24):3772-90
Designs with interim analysis
-
Grayling MJ, Wason JM, Mander AP. Group sequential designs for stepped-wedge cluster randomised trials. Clinical Trials. 2017;14(5):507-17
Sample size re-estimation
-
Grayling MJ, Mander AP, Wason JMS. Blinded and unblinded sample size reestimation procedures for stepped-wedge cluster randomized trials. Biometrical journal Biometrische Zeitschrift. 2018;60(5):903-16
Binary outcomes
-
Harrison LJ, Wang R. Power calculation for analyses of cross-sectional stepped-wedge cluster randomized trials with binary outcomes via generalized estimating equations. Statistics in Medicine. 2021:1-15
Design: Tools and software
Various practical resources have been produced for calculating sample size in SW-CRTs:
Excel tool when assuming equal correlation within clusters across all periods
Stata command “steppedwedge” when assuming equal correlation within clusters across all periods
-
Hemming K, Girling A. A menu-driven facility for power and detectable-difference calculations in stepped-wedge cluster-randomized trials. Stata Journal. 2014;14(2):363-80
R package “SWSamp” for using simulation based sample size calculations
-
Baio G. SWSamp: simulation-based sample size calculations for a stepped wedge trial (and more) 2016. Available from:
Online tool for comparing designs
-
Kasza J, Hemming K, Hooper R, Matthews J, Forbes A. Impact of non-uniform correlation structure on sample size and power in multiple-period cluster randomised trials. Statistical Methods in Medical Research. 2017:0962280217734981
R package “swCRTdesign” for the design and analysis of SW-CRTs
-
Hussey MA, Hughes JP. Design and analysis of stepped wedge cluster randomized trials. Contemporary Clinical Trials. 2007;28(2):182-91
Multi-arm SW-CRT design R code
-
Grayling M, Mander A, Wason J. Admissible multi-arm stepped-wedge cluster randomized trial designs. arXiv preprint arXiv:171003496. 2017
Design: Expected inter-period correlation coefficient and autocorrelation values
Sample size calculations for SW-CRTs require postulating a value for the inter-period correlation coefficient and a correlation structure for observations over time. In the following paper, Martin et al. have published some values:
-
Martin J, Girling A, Nirantharakumar K, Ryan R, Marshall T, Hemming K. Intra-cluster and inter-period correlation coefficients for cross-sectional cluster randomised controlled trials for type-2 diabetes in UK primary care. Trials. 2016;17(1):402
Design: Crossover CRTs
SW-CRTs are a modification of the crossover design. Recommendations about the design and sample size of cluster crossover trials can be found here:
-
Arnup SJ, McKenzie JE, Hemming K, Pilcher D, Forbes AB. Understanding the cluster randomised crossover design: a graphical illustration of the components of variation and a sample size tutorial. Trials. 2017;18(1):381
-
Reich NG, Milstone AM. Improving efficiency in cluster-randomized study design and implementation: taking advantage of a crossover. Open Access Journal of Clinical Trials. 2013;6:11-5
-
Reich NG, Myers JA, Obeng D, Milstone AM, Perl TM. Empirical Power and Sample Size Calculations for Cluster-Randomized and Cluster-Randomized Crossover Studies. PLOS ONE. 2012;7(4):e35564
-
Forbes AB, Akram M, Pilcher D, Cooper J, Bellomo R. Cluster randomised crossover trials with binary data and unbalanced cluster sizes: application to studies of near-universal interventions in intensive care. Clinical trials. 2015;12(1):34-44
-
Rietbergen C, Moerbeek M. The Design of Cluster Randomized Crossover Trials. Journal of Educational and Behavioral Statistics. 2011;36(4):472-90
-
Giraudeau B, Ravaud P, Donner A. Sample size calculation for cluster randomized cross‐over trials. Statistics in Medicine. 2008;27(27):5578-85
Analysis: Introduction
There are three main considerations in the analysis of SW-CRTS beyond those of the parallel, individually randomised trial:
-
Accounting for clustering. Like other CRTs, observations in the same cluster are likely to be more similar to one another than observations in different clusters and this needs to be accounted for in the analysis. Methods include using generalised linear mixed effect models, generalised estimating equations (GEE), and using cluster summary analyses (see resources for analysing CRTs for more information).
-
Accounting for changes in the outcome over time. In a SW-CRT, the intervention condition observations are on average later in time than the control observations, so the intervention effect is confounded with time and the analysis must account for this, either by adjusting for time or conditioning on time (known as a vertical analysis).
-
Accounting for changes in correlation over time. Observations collected close together in time are likely to be more similar to one another than observations collected further apart in time. This can be accounted for using generalised linear mixed effect models, or generalised estimating equations (GEE).
SW-CRTs provide information about the intervention from two types of comparisons. Most analyses combine both of these comparisons:
-
Horizontal, or within-cluster comparisons compare observations in the control condition to observations in the intervention condition within each cluster. These comparisons are confounded with time and the amount of information they can provide depends on the correlations over time within the clusters.
-
Vertical, or within-period comparisons compare observations in the control condition to observations in the intervention condition within each period of the study. Each of these comparisons is a randomised comparison and is not confounded with time; they are like CRTs contained within the SW-CRT.
This page provides resources to help with analysing a SW-CRT. It may also be useful to look at the resources for analysing CRTs, but here we focus on SW-CRTs. In particular, we present some general information, followed by resources for vertical analysis, generalised linear mixed models, generalised estimating equations, and permutation tests, and special considerations for time-to-event outcomes, modelling different aspects of the intervention effect, number of clusters required, causal inference methods, and the crossover design.
Analysis: General information
The following references provide general information on considerations for selecting an analysis method:
-
Hayes RJ, Moulton LH. Cluster randomised trials. Boca Raton: CRC Press; 2017
-
Barker D, McElduff P, D’Este C, Campbell MJ. Stepped wedge cluster randomised trials: a review of the statistical methodology used and available. BMC Med Res Methodol. 2016;16:69
-
Copas AJ, Lewis JJ, Thompson JA, Davey C, Baio G, Hargreaves JR. Designing a stepped wedge trial: three main designs, carry-over effects and randomisation approaches. Trials. 2015;16(1):352
-
Davey C, Hargreaves J, Thompson JA, Copas AJ, Beard E, Lewis JJ, et al. Analysis and reporting of stepped wedge randomised controlled trials: synthesis and critical appraisal of published studies, 2010 to 2014. Trials. 2015;16(1):358
Analysis: Vertical analysis
Some researchers have suggested using an analysis method that only utilises the vertical comparisons because these comparisons are not confounded with time. Combining the estimates from each vertical comparison must account for the correlation of observations in each period. The following references provide details of how this can be done:
-
Thompson JA, Davey C, Fielding K, Hargreaves JR, Hayes RJ. Robust analysis of stepped wedge trials using cluster-level summaries within periods. Statistics in medicine. 2018;37(16):2487-500
-
Matthews JNS, Forbes AB. Stepped wedge designs: insights from a design of experiments perspective. Statistics in Medicine. 2017;36(24):3772-90
-
Zhan et al (2016) The analysis of terminal endpoint events in stepped wedge designs. Statistics in Medicine. 2016;35(24):4413-4426
-
Hayes RJ, Moulton LH. Cluster randomised trials. Boca Raton: CRC Press; 2017
-
Granston TS. Addressing Issues with the Stepped Wedge Design of Cluster-Randomized Clinical Trials [Thesis] University of Washington2013.
Analysis: Generalised linear mixed models
Generalised linear mixed models combine vertical and horizontal comparisons. The following papers provide information on generalised linear mixed models for stepped wedge trials:
-
Li F, Hughes JP, Hemming K, Taljaard M, Melnick ER, Heagerty PJ. Mixed-effects models for the design and analysis of stepped wedge cluster randomized trials: An overview. Statistical Methods in Medical Research. 2021;30(2):612-639.
-
Kasza J, Forbes AB. Inference for the treatment effect in multiple-period cluster randomised trials when random effect correlation structure is misspecified. Statistical Methods in Medical Research. 2018:0962280218797151
-
Hemming K, Taljaard M, Forbes A. Modeling clustering and treatment effect heterogeneity in parallel and stepped‐wedge cluster randomized trials. Statistics in Medicine. 2018;37(6):883-98
-
Hemming K, Taljaard M, Forbes A. Analysis of cluster randomised stepped wedge trials with repeated cross-sectional samples. Trials. 2017;18(1):101
-
Thompson JA, Fielding KL, Davey C, Aiken AM, Hargreaves JR, Hayes RJ. Bias and inference from misspecified mixed‐effect models in stepped wedge trial analysis. Statistics in Medicine. 2017;36(23):3670-82
-
Hussey MA, Hughes JP. Design and analysis of stepped wedge cluster randomized trials. Contemporary Clinical Trials. 2007;28(2):182-91
Analysis: Generalised estimating equations
The following papers provide information on generalised estimating equations for stepped wedge trials:
-
Thompson JA, Hemming K, Forbes A, Fielding K, Hayes R. Comparison of small-sample standard-error corrections for generalised estimating equations in stepped wedge cluster randomised trials with a binary outcome: A simulation study. Statistical Methods in Medical Research. 2021;30(2):425-439
-
Ren Y, Hughes JP, Heagerty PJ. A simulation study of statistical approaches to data analysis in the stepped wedge design. Statistics in Biosciences. 2020;12(3):399-415
-
Scott JM, deCamp A, Juraska M, Fay MP, Gilbert PB. Finite-sample corrected generalized estimating equation of population average treatment effects in stepped wedge cluster randomized trials. Statistical Methods in Medical Research. 2017;26(2):583-97
Analysis: Permutation tests
Permutation methods can be used for SW-CRTs. The following provides an introduction to permutation tests:
-
Ernst MD. Permutation Methods: A Basis for Exact Inference. Statistical Science. 2004;19(4):676-85
The following is an R package, “permute”, for generating permutations in R:
The following is a stata command, “swpermute”, for conducting permutation tests for SW-CRTs:
-
Thompson J. SWPERMUTE: Stata module to compute Permutation tests for Stepped-Wedge Cluster-Randomised Trials Boston College Department of Economics2017.
The papers below provide details on permutation methods for SW-CRTs:
-
Wang R, De Gruttola V. The use of permutation tests for the analysis of parallel and stepped‐wedge cluster‐randomized trials. Statistics in Medicine. 2017;36(18):2831-43
-
Ji X, Fink G, Robyn PJ, Small DS. Randomization inference for stepped-wedge cluster-randomized trials: An application to community-based health insurance. Annals of Applied Statistics. 2017;11(1):1-20
Analysis: Randomisation based inference
The following paper provides information on randomisation-based inference:
-
Rabideau DJ, Wang R. Randomization-based inference for a marginal treatment effect in stepped wedge cluster randomized trials. Statistics in Medicine. 2021;40(20):4442-4456
Analysis: Time to event outcomes
-
Bellan SE, Pulliam JRC, Pearson CAB, Champredon D, Fox SJ, Skrip L, et al. Statistical power and validity of Ebola vaccine trials in Sierra Leone: a simulation study of trial design and analysis. The Lancet Infectious Diseases. 2015;15(6):703-10
-
Zhan Z, van den Heuvel ER, Doornbos PM, Burger H, Verberne CJ, Wiggers T, et al. Strengths and weaknesses of a stepped wedge cluster randomized design: its application in a colorectal cancer follow-up study. Journal of Clinical Epidemiology. 2014;67(4):454-61
-
Moulton LH, Golub JE, Durovni B, Cavalcante SC, Pacheco AG, Saraceni V, et al. Statistical design of THRio: a phased implementation clinic-randomized study of a tuberculosis preventive therapy intervention. Clinical Trials. 2007;4(2):190-9
Analysis: Modelling different aspects of the intervention effect
The SW-CRT design can allow modelling of a changing intervention effect:
-
Twisk JWR, Hoogendijk EO, Zwijsen SA, de Boer MR. Different methods to analyze stepped wedge trial designs revealed different aspects of intervention effects. Journal of Clinical Epidemiology. 2016;72:75-83
Analysis: Number of clusters required
-
Barker D, D’Este C, Campbell MJ, McElduff P. Minimum number of clusters and comparison of analysis methods for cross sectional stepped wedge cluster randomised trials with binary outcomes: A simulation study. Trials. 2017;18(1):119
Analysis: Causal inference
As with CRTs, SW-CRT it is possible to estimate a complier average causal effect:
-
Gruber JS, Arnold BF, Reygadas F, Hubbard AE, Colford JJM. Estimation of Treatment Efficacy With Complier Average Causal Effects (CACE) in a Randomized Stepped Wedge Trial. American Journal of Epidemiology. 2014;179(9):1134-42
Analysis: Crossover CRTs
SW-CRTs are a modification of the crossover design. Methods for the analysis of cross-over CRTs are described in the following:
-
Morgan KE, Forbes AB, Keogh RH, Jairath V, Kahan BC. Choosing appropriate analysis methods for cluster randomised cross‐over trials with a binary outcome. Statistics in Medicine. 2017;36(2):318-33
-
Arnup SJ, Forbes AB, Kahan BC, Morgan KE, McKenzie JE. Appropriate statistical methods were infrequently used in cluster-randomized crossover trials. J Clin Epidemiol. 2016;74:40-50
-
Forbes AB, Akram M, Pilcher D, Cooper J, Bellomo R. Cluster randomised crossover trials with binary data and unbalanced cluster sizes: application to studies of near-universal interventions in intensive care. Clinical trials (London, England). 2015;12(1):34-44
-
Parienti JJ, Kuss O. Cluster-crossover design: a method for limiting clusters level effect in community-intervention studies. Contemp Clin Trials. 2007;28(3):316-23
-
Turner RM, White IR, Croudace T. Analysis of cluster randomized cross‐over trial data: a comparison of methods. Statistics in Medicine. 2007;26(2):274-89
Reporting: Introduction
Adequate reporting of the trial results is essential to ensure transparency and reproducibility of findings. This page provides resources to help with the reporting of SW-CRTs.
Reporting: Guidelines
The Consolidated Standards of Reporting Trials (CONSORT) statement includes a checklist of items that should be included in the report of individually randomised trials. An extension for CRTs is available (see resources for the reporting of CRTs). There are many items in these statements that are applicable for a SW-CRT, and should be adhered to. The first discussion of reporting guidelines and the need for a set of recommendations for SW-CRTs can be found in the following paper:
-
Hemming K, Haines TP, Chilton PJ, Girling AJ, Lilford RJ. The stepped wedge cluster randomised trial: rationale, design, analysis, and reporting. British Medical Journal. 2015;350:h391
An extension to the CONSORT guidelines for SW-CRTs has recently been published. The protocol for this extension, and the explanation and elaboration paper can be found at:
-
Hemming K, Girling A, Haines T, R. L. Protocol: Consort extension to stepped wedge cluster randomised controlled trial Equator Network; 2014
-
Hemming K, Taljaard M, McKenzie JE, Hooper R, Copas A, Thompson JA, et al. Reporting of stepped wedge cluster randomised trials: extension of the CONSORT 2010 statement with explanation and elaboration. BMJ. 2018;363
Some of the key criteria for reporting are listed below:
Trial design
Description and schematic of trial design including definition of cluster, number of sequences, number of clusters randomised to each sequence, number of periods, and duration of time between each step. It should be reported whether the participants assessed in different periods from the same cluster are the same people, different people, or a mixture.
Sample size
The method of calculation and relevant parameters should be reported with sufficient detail so that the calculation is replicable. Any assumptions made about the correlations between outcomes of participants within the same cluster should be noted.
Methods of analysis
Statistical methods used to compare treatment conditions for primary and secondary outcomes including how time effects, clustering and repeated measures were taken into account.
Recruitment
Dates defining the recruitment and follow-up for participants, steps, set up of the intervention and deviations from planned dates.
Results
For each primary and secondary outcome, results for each treatment condition, and the estimated effect size and its precision (such as 95% confidence interval); any correlations and time effects estimated in the analysis.
The SW-CRT contains many unique design features. An accurate trial report should include the reporting of each of these key concepts, which can be found in detail below:
Effect of time on the design
Detailed description In a SW-CRT, clusters are randomised to different sequences which dictate at what date they will initiate the intervention. Observations collected under the control condition are therefore, on average, from an earlier calendar time than observations collected under the intervention condition.
Why this is important Changes external to the trial may create underlying secular trends. In addition, where participants are repeatedly assessed over the duration of the trial, participants might get sicker over the study. This means that time is a potential confounder. Analysis should allow for the confounding effect of time.
Recruitment of participants
Detailed description SW-CRTs make a series of measurements over time within each cluster. These repeated measurements can be on the same participants, different participants at each measurement, or a mixture of the same and different participants.
Why this is important Analysis (and consequently sample size calculations) should allow for the fact that data are not independent (i.e. repeated measures from the same cluster and possibly repeated measures on the same participants).
Sampling of observations
Detailed description SW-CRTs can take a complete enumeration of the cluster or take a random sample of individuals from the cluster. Furthermore, participants might be continuously recruited into the trial as they present; or all participants might be recruited at the beginning of the trial. Some trials don’t recruit participants at all, but rather use their data on outcomes only.
Why this is important Information on how observations were sampled is important to elicit risk of bias. Studies which take a complete enumeration are at lower risk of bias; as too are those studies which recruit all participants at a fixed point in time before randomisation has occurred; whereas those which continuously recruit are at higher risk of bias (due to potential for identification and recruitment biases).
Duration of exposure
Detailed description In SW-CRTs some or all of the clusters will be exposed to both the control and intervention condition. Individuals can either have a relatively short exposure to the intervention (surgical intervention) or long exposure (change in care home policy). Individuals might only be exposed to one of the treatment conditions, or both.
Why this is important Where duration of exposure is short it is unlikely that individuals will be exposed to both the control and intervention condition. Where the duration of exposure is long, it may be possible to sample the same individuals under the control condition and later under the intervention condition. In trials with long exposure and delayed assessment of outcome this could mean that participants recruited under the control condition become exposed to the intervention.
Continuous or discrete time measurements
Detailed description Observations may be accrued continuously in time (e.g., as patients present to an emergency department and provide measurements after a follow-up period); or in discrete time (e.g., a survey questionnaire may be implemented at several discrete points in time).
Why this is important Where observations are accrued in continuous time, time will be measured as a continuous variable; where observations are accrued in discrete time, time will be measured as a categorical variable. This can have implications for choice of analysis.
Reporting: Current standard
Previous systematic reviews have highlighted the poor level of reporting in SW-CRTs – particularly in the area of sample size, analysis methods, and ethical conduct. The below resources indicate the quality of reporting in specific areas.
-
Martin J, Taljaard M, Girling A, Hemming K. Systematic review finds major deficiencies in sample size methodology and reporting for stepped-wedge cluster randomised trials. BMJ Open. 2016;6(2)
-
Taljaard M, Hemming K, Shah L, Giraudeau B, Grimshaw JM, Weijer C. Inadequacy of ethical conduct and reporting of stepped wedge cluster randomized trials: Results from a systematic review. Clinical Trials. 2017;14(4):333-41
-
Grayling MJ, Wason JMS, Mander AP. Stepped wedge cluster randomized controlled trial designs: a review of reporting quality and design features. Trials. 2017;18(1):33
-
Beard E, Lewis JJ, Copas A, Davey C, Osrin D, Baio G, et al. Stepped wedge randomised controlled trials: systematic review of studies published between 2010 and 2014. Trials. 2015;16:353
-
Brown CA, Lilford RJ. The stepped wedge trial design: a systematic review. BMC Medical Research Methodology. 2006;6(1):54